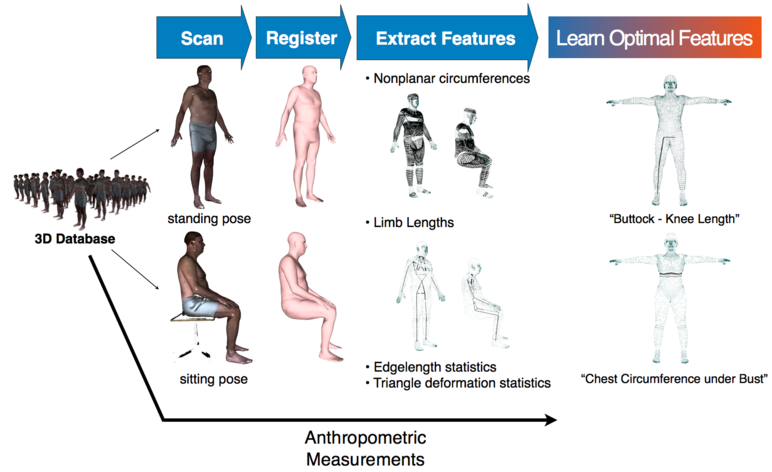
We predict anthropometric measurements from multiple poses using a model-based approach. We start with a database of 3D scans in multiple poses (standing, sitting) with corresponding anthropometric measurements (CAESAR). First, we register the scans using prior knowledge about human body shape (a human body model). Second, we extract shape features. We consider local features, such as body circumferences and limb lengths, as well as global features, such as statistics on edge lengths and triangle deformations of the registered meshes. Third, we learn optimal features for predicting each measurement.
Extracting anthropometric or tailoring measurements from 3D human body scans is important for applications such as virtual try-on, custon clothing, and online sizing. Existing commercial solutions identify anatomical landmarks on high-resolution 3D scans and then compute distances or circumferences on the scan. Landmark detection is sensitive to acquisition noise (e.g. holes) and these methods require subjects to adopt a specific pose. In contrast, we propose a solution that we call model-based anthropometry. We fit a deformable 3D body model to scan data in one or more poses; this model-based fitting is robust to scan noise. This brings the scan into registration iwth a database of registered body scans. Then, we extract features from the registered model (rather than from the scan); these include, limb lengths, circumferences, and statistical features of global shape. Finally, we learn a mapping from these features to measurements using regularized linear regression. We perform an extensive evaluation using the CAESAR dataset and demonstrate that the accuracy of our method outperforms state-of-art methods.
